AI-driven search is reshaping how content is discovered. Google’s AI Overviews (AIOs), ChatGPT, and Gemini now generate answers instead of ranking pages, and organic traffic is declining. Marketing leaders are under pressure to adapt—but how?
For B2B SaaS companies, this shift isn’t just a search visibility problem. It’s a pipeline risk. Marketing leaders are under pressure to explain why organic traffic is declining, why competitors are being referenced in AI-generated answers, and what needs to change.
SEO isn’t dead, but rankings alone no longer guarantee visibility. AI models prioritize structured, authoritative content, making traditional keyword strategies less effective. Without strong SEO foundations, GEO doesn’t work.
This guide breaks down what’s changing, what still matters, and how to optimize for both SERPs and AI-generated search—so your content stays visible, no matter how search evolves.
Understanding SEO vs GEO
What is traditional SEO?
Search Engine Optimization (SEO) is the foundation of digital marketing for traditional search engines like Google and Bing. It involves optimizing web pages to rank higher on search engine results pages (SERPs) by improving relevance, authority, and user experience.
Key elements of traditional SEO:
- Keyword optimization: Matching user search queries with relevant content
- Backlinks: Earning links from authoritative sites to build credibility
- Technical SEO: Enhancing site speed, structured data, and mobile-friendliness
- User experience (UX): Improving engagement metrics like bounce rate and click-through rate
Traditional SEO relies heavily on ranking factors determined by search engine algorithms. Studies show that Google’s core ranking systems prioritize E-E-A-T (Experience, Expertise, Authoritativeness, and Trustworthiness), meaning that credibility plays a major role in visibility.
However, the landscape is shifting. With the rise of AI-driven search engines, traditional SEO alone is no longer enough.
What is Generative Engine Optimization (GEO)?
Generative Engine Optimization (GEO) is the practice of optimizing content for AI-driven search engines like Google’s AI Overviews (AIO), Perplexity, and ChatGPT. Instead of ranking web pages, these AI models generate responses by synthesizing information from multiple sources.
How GEO differs from traditional SEO:
- Entity-based optimization: AI models recognize entities (people, brands, products) rather than relying solely on keywords
- Structured content: AI favors clear, FAQ-style responses, bullet points, and schema markup
- Citations & trust signals: AI-generated results often reference trusted sources, making accurate citations and brand authority essential
- Conversational intent: AI search tools prioritize contextual relevance and natural language processing (NLP)
According to a 2024 study comparing ChatGPT, Gemini, and Perplexity, AI search models prioritize structured data and factual accuracy over traditional ranking signals like backlinks.
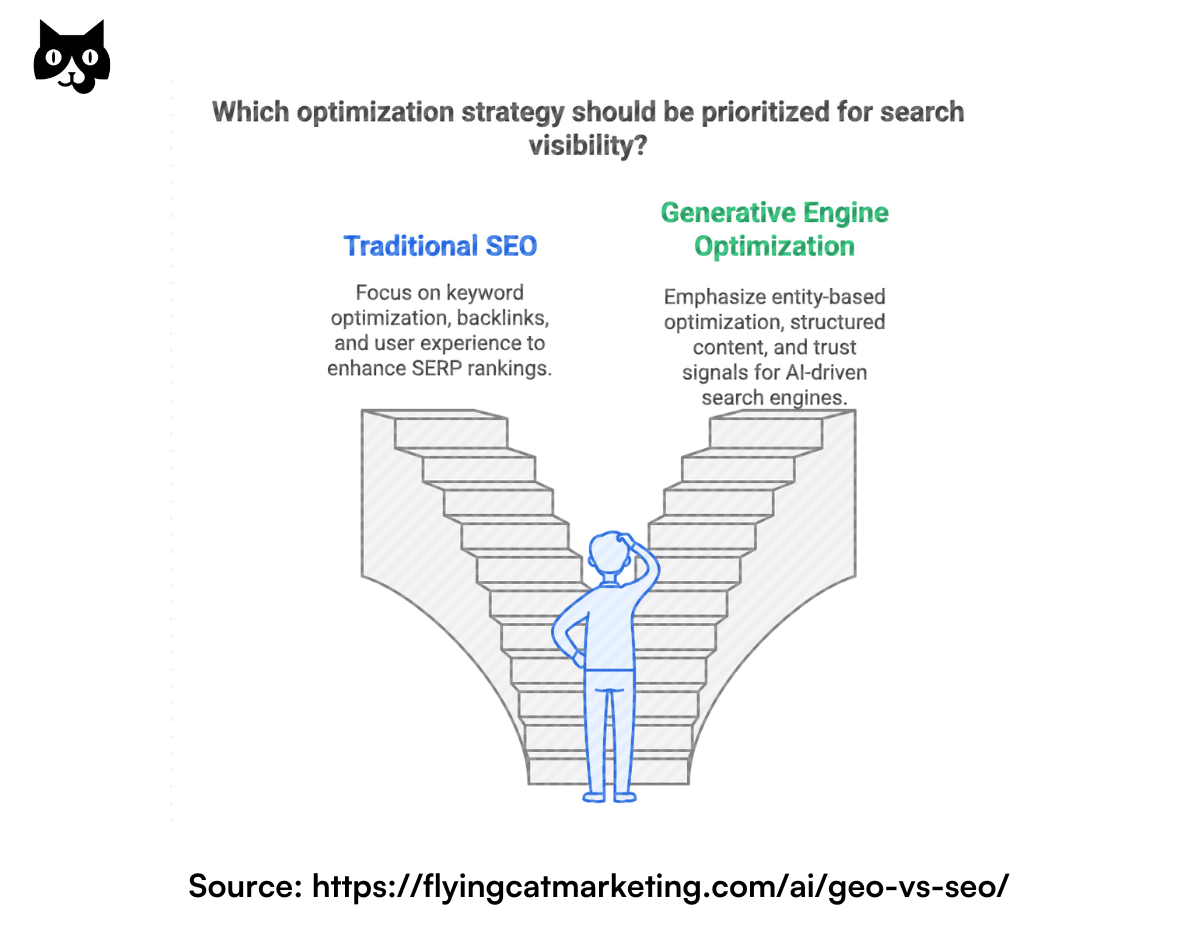
Key differences between SEO and GEO
Factor | SEO | GEO |
Search engines | Google, Bing | AI-driven search engines (AIOs, ChatGPT, Gemini) |
Optimization focus | Keywords, backlinks, user experience | AI algorithms, structured responses, entity recognition |
Ranking factors | Click-through rate, content quality, authority | Accuracy, citations, structured content |
GEO shifts the focus from ranking in SERPs to being referenced by AI-generated responses. Marketers who fail to adapt risk losing visibility in the AI-driven search landscape.
How AI search engines change content optimization
AI-driven search engines like AIOs, Gemini, and ChatGPT are changing the way content is surfaced and consumed. Unlike traditional search engines that rely on ranking web pages, these AI models generate responses by pulling information from multiple sources. This shift has major implications for content optimization.
AI systems parse information
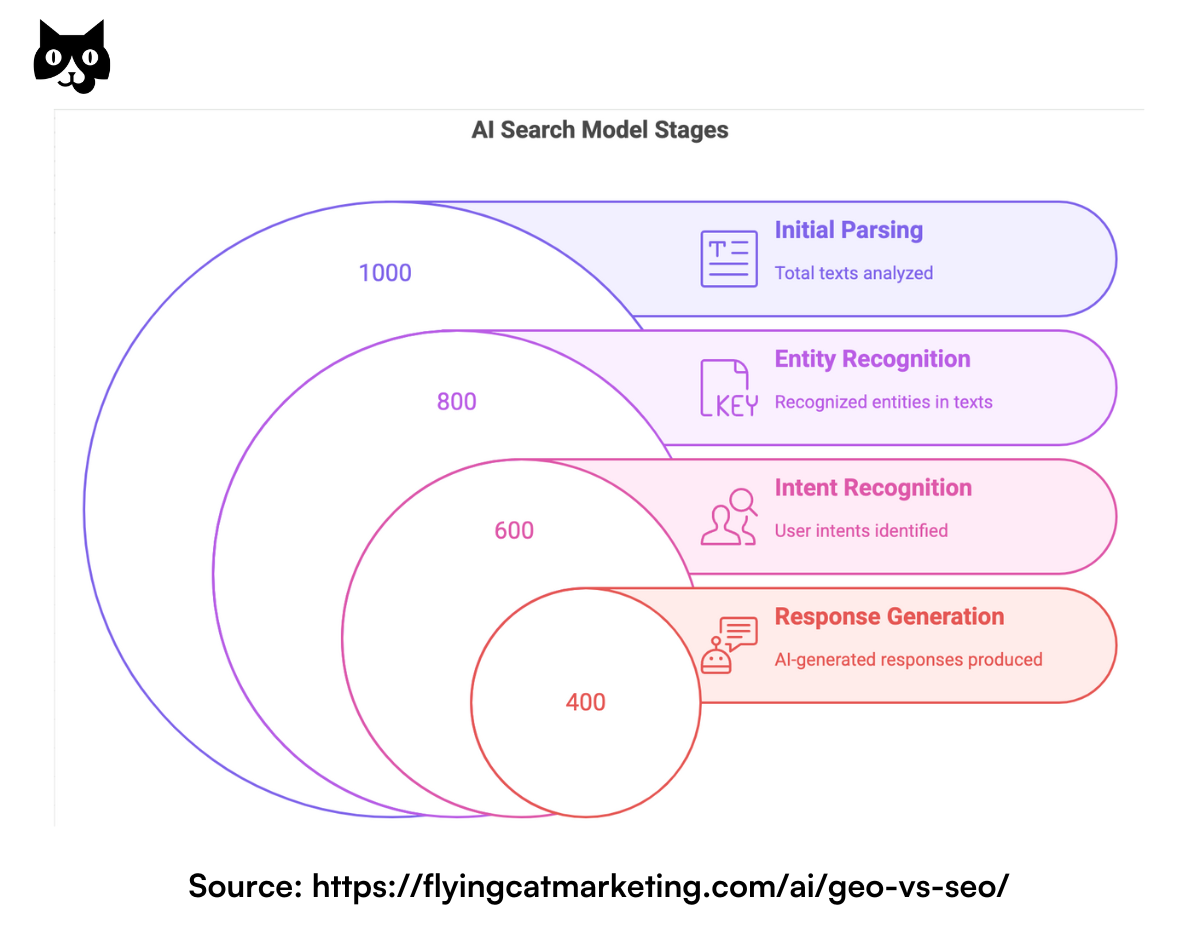
Parsing is the process by which an AI system analyzes and structures text to extract meaning. Unlike traditional search engines that scan for keywords and backlinks, AI-driven models use Natural Language Understanding (NLU) to interpret content contextually rather than just matching words.
Natural Language Understanding (NLU) is a branch of Natural Language Processing (NLP) that focuses on comprehending the meaning of text rather than just processing it. Traditional NLP methods rely on pattern recognition and statistical models, whereas NLU aims to extract context, search intent, and relationships between entities.
Technically, NLU involves:
- Syntactic parsing: Breaking down sentences into grammatical structures (nouns, verbs, objects, etc.)
- Semantic parsing: Understanding the meaning of words in context
- Entity recognition; Identifying specific entities such as brands, people, and locations
- Intent recognition; Determining what the user is asking or searching for
In simpler terms, traditional search engines work like a librarian using an index: they retrieve pages based on matching keywords. AI-driven search engines work more like a research assistant who understands the topic: they summarize, interpret, and connect ideas rather than just retrieving matching text.
AI search models, such as AIO (AI Overviews), ChatGPT, and Claude, do not rank pages in a traditional sense. Instead, they:
- Generate responses by summarizing multiple sources. Rather than displaying a list of ranked links, AI engines synthesize content from various websites, prioritizing concise, well-structured, and factually accurate information. This means web pages are not surfaced individually—content is blended into AI-generated answers, and only a few sources are cited, meaning brand visibility depends on appearing in AI-generated summaries.
- Prioritize entity-based search over keyword-based search. Instead of just matching keywords, AI models recognize entities—people, places, brands, products, and concepts. For example, in traditional SEO, ranking for “best CRM software” requires optimizing for that exact phrase. In AI-driven search, AI recognizes Salesforce, HubSpot, and Pipedrive as CRMs, pulling context from multiple sources rather than just matching the phrase “best CRM software.” This means SEO strategies must shift from keyword stuffing to entity optimization.
- Prefer structured content for easy extraction. AI models extract information more efficiently when content is formatted in a structured way. They favor clear headings and subheadings, bullet points and numbered lists, FAQs, and concise summaries.
A 2024 study on ChatGPT, Gemini, and Perplexity found that structured content is 50 percent more likely to be referenced in AI-generated search results than long-form, unstructured text.
AI-driven search changes how content is discovered. If content is not structured, entity-rich, and contextually clear, it may never appear in AI-generated results.
GEO requires even more citations and trustworthiness at a page level
AI-driven search engines do not rank pages the way traditional search engines do. Instead, they extract and synthesize information from multiple sources, referencing only a select few. This changes how trust and credibility work in search visibility.
Rather than relying on backlinks as a primary ranking factor, generative engines prioritize factual accuracy, citations, and structured content.
A study from Princeton University and IIT Delhi measured how often different types of content appeared in AI-generated responses.
It found that structured citations, quotations from authoritative sources, and the inclusion of verifiable statistics significantly increased the likelihood of being referenced.
Websites that optimized for these factors saw up to a 40 percent increase in visibility compared to those relying on traditional SEO techniques.
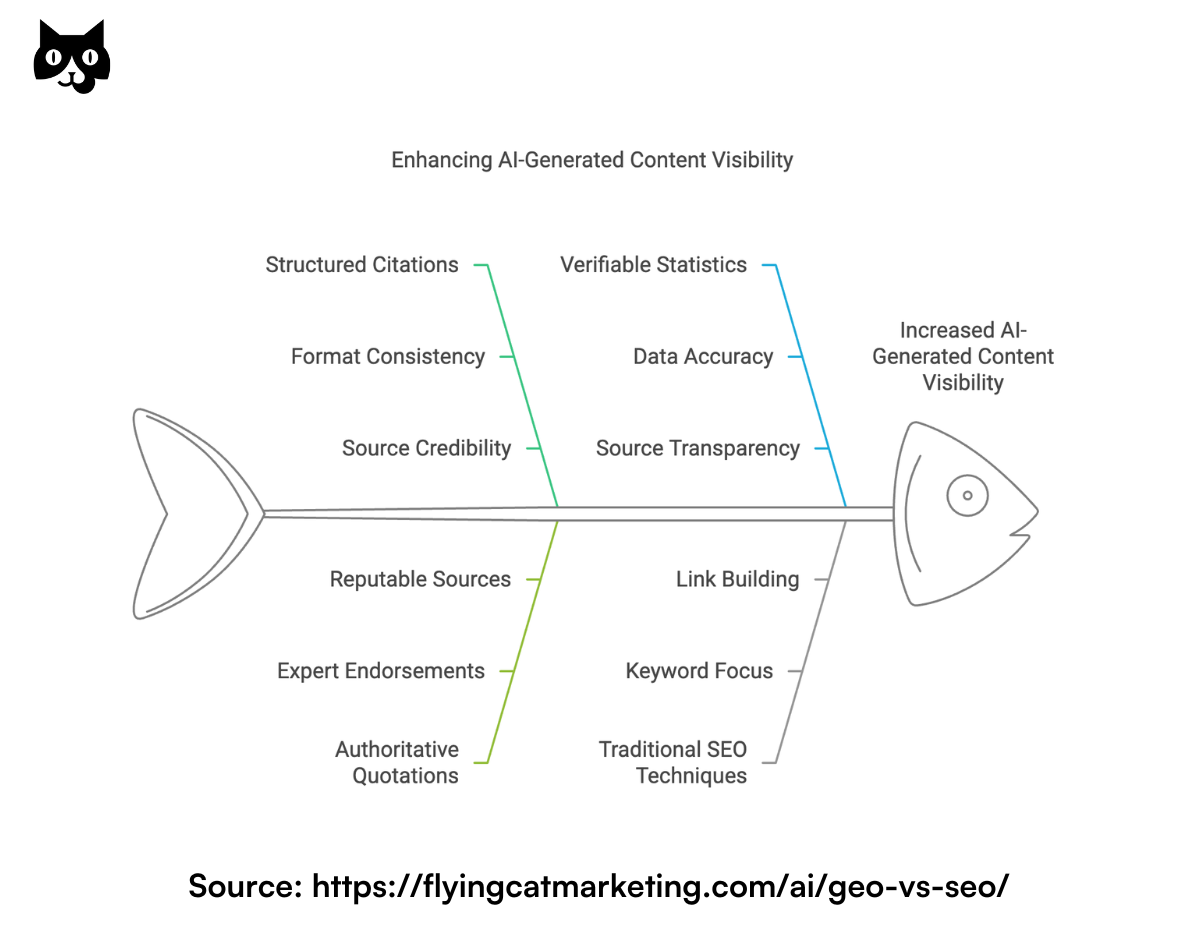
This shift raises questions about how Google’s E-E-A-T framework (Experience, Expertise, Authoritativeness, and Trustworthiness) applies in an AI-driven search landscape. E-E-A-T has been a guiding principle for ranking content in traditional search, emphasizing the credibility of a website or author.
But generative engines evaluate trustworthiness differently. Instead of assessing a website’s overall authority, they determine whether individual content pieces contain clear, verifiable, and structured information. Even highly authoritative websites may not be referenced if their content is ambiguous, lacks citations, or is not formatted for AI extraction.
The same Princeton study introduced GEO-bench, a benchmark designed to measure visibility across AI-generated search results.
The results confirmed that optimization strategies for AI-driven search engines must account for different factors than traditional SEO.
Websites that included structured citations, direct quotations, and clear sourcing were more frequently referenced. The study also found that AI models were 40 percent more likely to include content that aligned with widely accepted expert consensus, reinforcing the importance of trust signals beyond simple authority scores.
To build credibility in an AI-driven search landscape, content should:
- Provide clear citations to authoritative sources rather than making unsupported claims
- Use structured data, such as schema markup and FAQ-style formatting, to reinforce clarity
- Ensure that information aligns with expert consensus rather than presenting unverified opinions
- Maintain consistency across multiple platforms to strengthen entity recognition
- Prioritize factual accuracy over speculation
AI-driven search engines do not return results based on traditional rankings. Instead, they select content that they determine to be the most reliable and well-structured for extraction. This makes citations and verifiability critical for maintaining visibility in AI-generated responses.
Keywords don’t exist in an AI landscape
Keyword research has long been a cornerstone of SEO. Every strategy begins with identifying the exact words and phrases users type into search engines and then optimizing content to match those queries. In AI-driven search, this approach falls apart.
Large language models don’t rely on keyword matching to retrieve information. They interpret meaning. Instead of ranking results based on specific words, they analyze the context, intent, and relationships between concepts. This means that keywords, as we’ve known them, might disappear entirely as a tool for optimization.
In traditional search, you could track keyword volume, identify the highest-performing variations, and optimize pages accordingly. In an AI-driven search landscape, there is no way to see how users phrase their queries. There is no search volume data, no keyword list, no ranking report that tells you which words to target.
That’s because a single user intent can be expressed in an infinite number of ways, and AI models are designed to understand and respond to all of them.
Take a B2B example. A marketing leader at a SaaS company might be looking for guidance on reducing customer churn. In a traditional search engine, they might type:
- “How to reduce SaaS churn”
- “Best strategies for retaining SaaS customers”
- “Lower churn rate in subscription businesses”
Each of these searches would return slightly different results, and a content strategist would optimize pages accordingly, making sure their article contained the right mix of high-intent keywords.
In an AI-driven search engine, the same user might phrase their query in ways that traditional keyword tools would never capture:
- “Customers keep canceling my software—what should I do?”
- “I need to improve retention for a SaaS platform with a high churn rate”
- “Why do users stop paying for software, and how do I fix it?”
Every one of these queries is unique, but the intent is the same. A traditional search engine would treat them as separate searches, ranking pages based on how well they match specific words. An AI-driven search engine recognizes that all of these queries point to the same fundamental question and generates a response accordingly.
This changes everything. Without keyword data, there is no way to optimize for every possible query variation. Instead, content must be created to serve an intent, not match a phrase.
AI-driven search engines don’t need to see an exact keyword to surface a relevant answer. They extract the underlying meaning and pull from sources that best address it.
For content marketers, this means shifting from keyword-driven strategies to intent-driven strategies. Instead of chasing rankings for specific phrases, the focus must be on creating content that thoroughly answers the core questions users have, no matter how they phrase them. Content that delivers clear, structured, and insightful information will be surfaced—regardless of whether it contains a specific keyword or not.
This is the moment to rethink how content is planned, optimized, and measured. AI-driven search engines don’t work like Google’s traditional algorithm, and holding onto old strategies will only lead to diminishing visibility. The companies that adapt now—by focusing on depth, clarity, and intent—will be the ones that remain visible as AI search takes over.
Content creation for both SEO and GEO
SEO and GEO require different optimization methods, but they are not competing strategies. The shift to AI-driven search does not mean abandoning traditional SEO. It means adapting content so that it remains visible in both search engine results pages (SERPs) and AI-generated answers.
Optimizing for traditional search means ensuring that content is indexed, ranks well, and satisfies user queries. Optimizing for AI-driven search means structuring content so that AI models can extract, interpret, and reference it in generated responses. A future-proof content strategy must account for both.
To align with how AI models process and generate content, focus on:
- Optimizing for SERPs and AI-generated answers. Content should still follow best practices for traditional rankings, including technical SEO, internal linking, and engagement metrics. At the same time, it should be structured for AI models to extract and synthesize easily.
- Using high-quality content that is concise and fact-driven. AI-generated search models prioritize factual accuracy and clear attribution. Long, unstructured content with speculative claims is less likely to be cited.
- Leveraging structured data. Schema markup, FAQ sections, and tables make content easier for AI to process. Studies on AI-driven search behavior have shown that structured responses increase the likelihood of being referenced in AI-generated answers.
The goal is to make content both searchable and extractable. Traditional search engines surface results based on ranking factors, while AI models synthesize information from multiple sources. The best strategy is one that accounts for both, ensuring visibility no matter how search evolves.
GEO-friendly formatting strategies
AI-driven search engines do not extract information the way traditional search engines do. Instead of ranking pages based on backlinks and keyword relevance, they look for content that is structured, scannable, and easy to synthesize. Formatting plays a critical role in determining whether content is selected for AI-generated responses.
To optimize for GEO, content should:
- Use structured responses. AI models process information more efficiently when it is presented in a clear, predictable format. Short paragraphs, bullet points, tables, and FAQ sections increase the likelihood of content being referenced. Dense, unstructured text is harder for AI to extract from and less likely to appear in generative search results.
- Enhance entity recognition. AI does not rely on exact keyword matching. Instead, it identifies entities (e.g. people, brands, and concepts) and connects them to related information. Clearly naming products, companies, and key terms helps AI engines understand relationships between topics. Ambiguous or vague language makes it harder for AI to process content effectively.
- Use citations. AI models prioritize verifiable information. Citing authoritative sources signals credibility and increases the likelihood of content being included in AI-generated responses. The presence of structured citations has been shown to improve visibility in generative search models.
Formatting is no longer just a matter of readability. It is a key factor in whether AI-driven search engines can recognize, extract, and attribute content. As AI continues to shape how users receive information, optimizing for structured extraction will be just as important as optimizing for rankings.
SEO techniques that still apply to GEO
Generative Engine Optimization (GEO) does not replace SEO, it builds on it. Without strong SEO foundations, GEO simply does not work. AI-driven search engines still rely on structured, authoritative, and well-optimized content. The difference is in how that content is processed and surfaced.
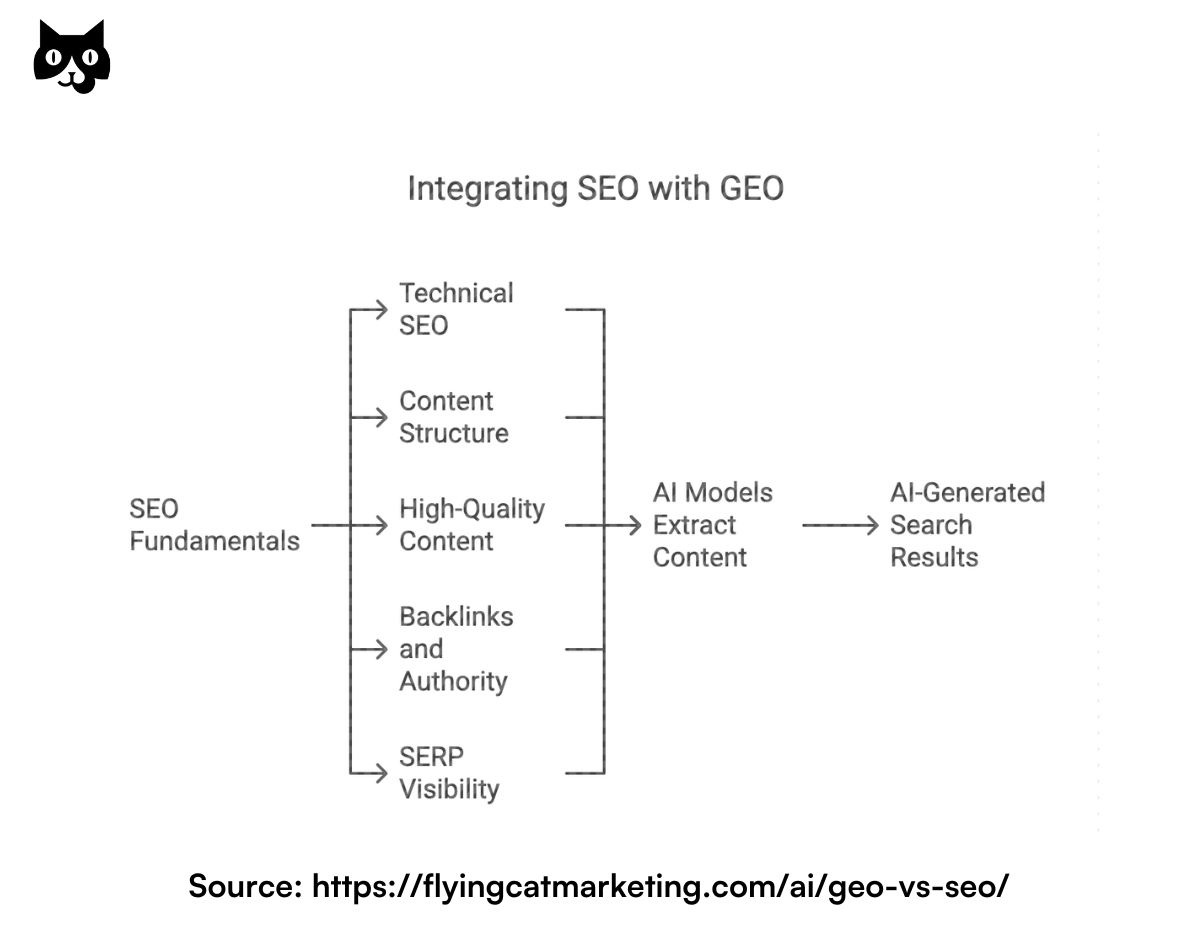
The core SEO fundamentals that remain essential for GEO include:
- Technical SEO. AI models extract and synthesize content, but they still need to find and interpret it correctly. A site that is not properly crawled and indexed will not appear in AI-generated search results. Ensuring clean site architecture, structured data implementation, and fast-loading pages remains critical.
- Content structure and clarity. AI-driven search engines process information contextually, but they prioritize structured and well-organized content. Content that follows clear heading hierarchies, uses schema markup, and presents information in scannable formats (such as bullet points and tables) is easier for AI models to extract and reference.
- High-quality, fact-driven content. AI models prioritize accuracy and reliability. Content that lacks depth, provides vague answers, or does not include supporting evidence will not be cited in AI-generated responses. GEO depends on the same principles as traditional SEO: expertise, thoroughness, and relevance.
- Backlinks and authority building. While AI-driven search engines do not rely on backlinks in the same way traditional search engines do, authority still matters. Sites that consistently earn links from authoritative sources and are frequently cited across the web are more likely to be referenced in AI-generated results.
- SERP visibility. AI search is growing, but traditional search is not going away. Optimizing for organic rankings still drives traffic and reinforces brand authority. Many AI-generated answers are sourced from high-ranking traditional search results, meaning strong SEO performance increases the likelihood of being included in generative responses.
GEO is not an isolated practice. It’s an extension of SEO. Without strong technical foundations, structured content, and clear authority signals, AI-driven search engines will not recognize or reference a website’s content. The best GEO strategy starts with getting SEO right.
Actionable GEO-SEO Checklist
A successful GEO strategy starts with strong SEO foundations and adapts to the way AI-driven search engines process content. Use this checklist to ensure your content remains visible in both traditional and AI-generated search results.
Understand and align with AI-driven search intent
☑ Identify key topics rather than relying on exact-match keywords
☑ Focus on user intent—what problem does the user need to solve?
☑ Optimize content for conversational and question-based queries
Structure content for AI extraction
☑ Use FAQs, bullet points, tables, and clear subheadings to improve readability
☑ Implement schema markup (FAQ, How-To, Article) to help AI models extract information
☑ Keep answers concise, fact-driven, and verifiable
Optimize for entity recognition and citations
☑ Clearly define brands, products, and key concepts
☑ Provide structured citations to authoritative sources
☑ Align content with widely accepted expert consensus
Monitor AI-driven search engines for visibility
☑ Track how content appears in AIOs, ChatGPT, and Perplexity responses
☑ Test prompts and variations to see how AI-generated answers change
☑ Adjust formatting and clarity based on how AI tools extract information
Maintain strong SEO fundamentals
☑ Ensure clean technical SEO (crawling, indexing, site speed)
☑ Continue building authority through backlinks and mentions
☑ Optimize for SERP visibility, as traditional search still plays a role
A GEO strategy is only as strong as the SEO foundation it is built on. AI-driven search engines favor structured, authoritative, and well-optimized content—getting these elements right ensures long-term visibility in both traditional and AI-generated search.
The future of search is hybrid
SEO and GEO are not opposing forces. They are part of the same evolving search landscape, each shaping how content is discovered and surfaced. Traditional SEO ensures that content is crawled, indexed, and ranked in search engine results pages. GEO ensures that content is recognized, extracted, and referenced in AI-generated answers.
The key differences come down to how search engines process information:
- SEO ranks pages. Traditional search engines evaluate keywords, backlinks, and engagement metrics to determine rankings.
- GEO extracts and synthesizes. AI-driven search engines pull from multiple sources and prioritize structured, fact-driven content over keyword-based relevance.
But the foundations remain the same:
- Both prioritize authority and trust. Whether ranking in SERPs or being cited in AI-generated responses, content must be accurate, well-structured, and backed by authoritative sources.
- Both require strong technical optimization. A poorly optimized site will not rank in Google, nor will it be surfaced by AI-driven search engines.
- Both depend on clear, structured content. Traditional search engines reward readability and relevance. AI search models favor structured formats, entity recognition, and well-sourced information.
As AI search grows, companies that focus solely on keyword rankings will lose visibility. But those that embrace both SEO and GEO strategies will remain discoverable—whether through a top organic ranking or an AI-generated answer. The future of search is not either-or. It’s both.